This blog post is written by Janet Butler, Director, TheWorkingSolution.com.
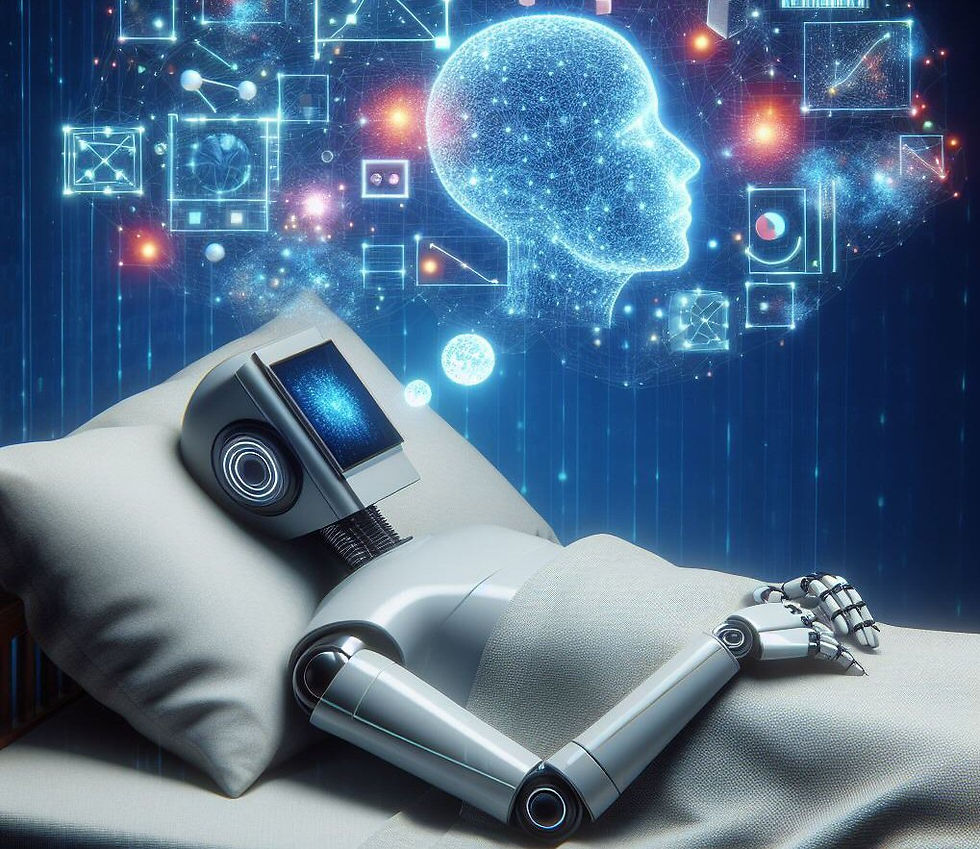
Hallucinations occur when AI algorithms generate outputs that do not accurately reflect the input data. That is answers generated by OpenAI ChatGBT and other AI chatbots, based on Large Language Module (LLM) training data, produce answers that may sound plausible, but are actually factually incorrect, not aligned with reality and don’t exist, hence the term hallucination!
One of the most notable examples includes that from the Google Bard chatbot which incorrectly claimed that the James Webb Space Telescope had captured the World’s first images of a planet outside our solar system!
Although, many of the initial ChapGPT glitches have been able to be fixed, as they were caused by incomplete training data, ‘hallucinations’ still exist and have wider implications when accurate answers are critical in the health, autonomous vehicle drive and financial sectors where inaccuracies can have serious consequences.
Therefore, researchers and developers are now actively working to minimize the occurrence of AI hallucinations through rigorous testing, validation and ongoing monitoring of AI systems.
In healthcare, for example, AI hallucinations could result in misdiagnosis or incorrect treatment recommendations, potentially jeopardizing patient safety and well-being. If an AI system generates hallucinatory results in medical imaging analysis or diagnostic decision-making, it could lead to incorrect medical interventions.
In the context of autonomous vehicles, AI hallucinations could result in misinterpretation of the surrounding environment, leading to incorrect driving decisions and potentially causing accidents or traffic disruptions. This poses significant risks to public safety and underscores the importance of accurate and reliable AI systems in the field of autonomous transportation.
In the financial sector, AI systems are utilized for fraud detection, risk assessment, and trading algorithms. If an AI system experiences hallucinations, it could generate erroneous predictions, leading to financial losses, incorrect risk assessment, or failure to detect fraudulent activities. This can have severe financial implications for businesses and individuals relying on AI-powered financial services.
Moreover, in sensitive decision-making processes, such as criminal justice, employment screening, or loan approvals, AI hallucinations could lead to biased or unfair outcomes, perpetuating existing societal inequalities and injustices.
The potential negative consequences of AI hallucinations underline the critical importance of ensuring the reliability and robustness of AI systems across various applications. Developers and researchers must consider and address the risks associated with AI hallucinations through rigorous testing, validation procedures, and ongoing monitoring to mitigate the impact of these anomalies on real-world scenarios.
Verifying AI hallucinations is a complex task that requires careful consideration and a multi-faceted approach. AI hallucinations can be defined as instances where an AI system generates outputs that are not consistent with the expected or desired behaviour. Verifying the presence of AI hallucinations involves understanding the underlying causes, assessing the impact, and identifying strategies to mitigate or prevent them.
Here are some approaches to verifying AI hallucinations:
1. Establishing Baseline Performance: The first step in verifying AI hallucinations is to establish a baseline performance for the AI system. This involves testing the system under normal operating conditions and validating its outputs against known, expected results. By establishing a baseline, deviations from expected behaviour can be more easily identified and assessed.
2. Input Validation and Data Integrity: AI systems rely on input data to make decisions and generate outputs. Verifying the integrity and validity of the input data is crucial in preventing and identifying hallucinations. Implementing robust data validation processes, such as anomaly detection and data integrity checks, can help ensure that the AI system is processing accurate and reliable input.
3. Human-in-the-Loop Verification: Introducing human oversight and verification into the AI system's decision-making process can be an effective way to identify hallucinations. By involving human experts who can review and validate the AI system's outputs, potential hallucinations can be flagged and addressed in a timely manner.
4. Cross-Validation and Ensemble Methods: Employing cross-validation techniques and ensemble methods can help identify discrepancies and inconsistencies in the AI system's outputs. By comparing the results of multiple models or algorithms, deviations from expected behaviour can be more readily detected, signalling potential hallucinations.
5. Real-time Monitoring and Feedback Loops: Implementing real-time monitoring mechanisms and feedback loops can enable the continuous assessment of the AI system's performance. By tracking the system's output and comparing it to expected outcomes, potential hallucinations can be identified in a timely manner, allowing for corrective actions to be taken.
6. Stress Testing and Adversarial Examples: Subjecting the AI system to stress testing and adversarial examples can help reveal vulnerabilities and potential sources of hallucinations. By intentionally exposing the system to challenging scenarios and input data, weaknesses in its decision-making processes can be uncovered, enabling the identification and mitigation of potential hallucinations.
7. External Audit and Peer Review: Seeking external audit and peer review of the AI system's outputs can provide an independent assessment of its performance. Engaging third-party experts and peers to evaluate the system's outputs can help identify potential hallucinations and provide valuable insights into their underlying causes.
8. Ethical and Regulatory Frameworks: Leveraging ethical and regulatory frameworks can also play a crucial role in verifying AI hallucinations. By adhering to guidelines and standards that promote transparency, accountability, and fairness in AI systems, the risk of hallucinations can be mitigated, and their presence can be more effectively handled.
It's important to note that verifying AI hallucinations is an ongoing and iterative process that requires collaboration among experts in AI, data science, ethics, and domain-specific fields. By implementing a combination of the aforementioned approaches and continuously refining verification methodologies, the presence of AI hallucinations can be identified, addressed, and ultimately minimized, contributing to the responsible and reliable deployment of AI technologies.